In the fast-paced world of e-commerce, personalized shopping experiences have become the cornerstone of customer satisfaction and loyalty.
Data labeling, the process of annotating data with relevant tags, plays a pivotal role in transforming e-commerce by enabling businesses to understand their customers better.
In this article, we’ll explore how data labeling is revolutionizing the e-commerce landscape and driving personalized shopping experiences to new heights.
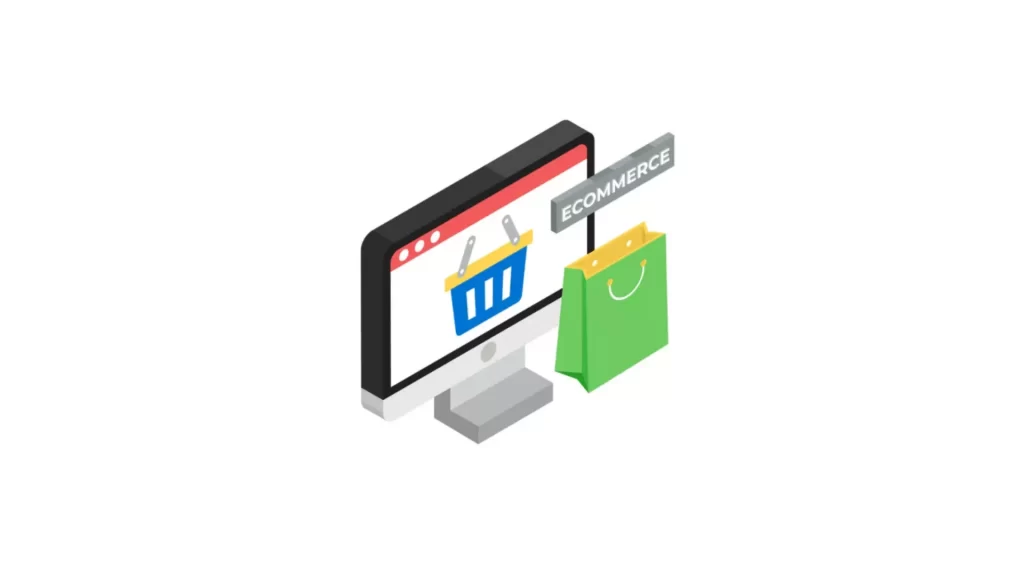
1. Understanding Customer Preferences with Data Labeling
Data labeling empowers e-commerce platforms to gain deep insights into customer preferences and behaviors. By accurately tagging product attributes, customer sentiments, and purchasing patterns, businesses can create comprehensive customer profiles.
This valuable data enables e-commerce platforms to deliver personalized product recommendations and targeted marketing strategies, elevating the overall shopping experience.
2. Enhancing Product Search and Discovery
Data labeling ensures product data is appropriately categorized and tagged, making search engines more efficient and accurate.
Advanced data labeling techniques, such as image and text annotation, enable e-commerce search algorithms to recognize objects, extract features, and understand natural language queries. This translates to quicker, more relevant search results, ultimately leading to increased customer satisfaction and conversion rates.
3. Personalization for Customer Retention
With data labelling tool, e-commerce platforms can develop recommendation systems that offer personalized shopping experiences. By leveraging historical purchase data and customer behavior, these systems suggest products tailored to each individual’s preferences.
The result is a higher likelihood of repeat purchases, increased customer retention, and improved brand loyalty.
4. Overcoming Challenges with Data Labeling
As the demand for personalized experiences grows, so does the complexity of data labeling. E-commerce businesses face challenges in accurately labeling diverse datasets, especially unstructured data like user-generated reviews and images.
Additionally, addressing privacy concerns while collecting and labeling customer data is essential to maintain trust and compliance.
Innovative Solutions for Data Labeling in E-commerce
1. Semi-Supervised Learning
Semi-supervised learning techniques combine labeled and unlabeled data to train models effectively. This approach allows e-commerce businesses to make the most of available labeled data while reducing labeling costs and increasing the efficiency of their AI models.
2. Active Learning
Active learning enables e-commerce platforms to identify the most informative data samples that require labeling. By focusing human annotators’ efforts on challenging data points, businesses can achieve accurate models with minimal annotation efforts.
3. Crowdsourcing Data Labeling
Crowdsourcing platforms offer a scalable and cost-effective solution for e-commerce businesses to access a large pool of human labelers. These crowd workers can efficiently label vast amounts of data, accelerating model training and improving overall performance.
Conclusion
Data labeling is a game-changer for e-commerce and personalization, providing businesses with valuable insights into customer preferences, enhancing product search capabilities, and driving customer retention.
By leveraging innovative solutions like semi-supervised learning, active learning, and crowdsourcing, e-commerce platforms can overcome the challenges associated with data labeling and deliver exceptional personalized shopping experiences.
In the competitive landscape of e-commerce, mastering the art of data labeling can be the key to gaining a competitive edge, maximizing customer satisfaction, and fostering long-lasting customer relationships.
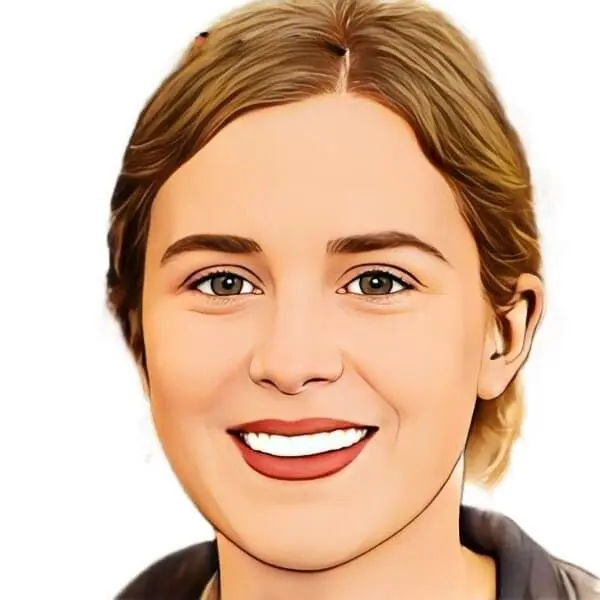
I’m Aurelia Brown! I blog about tech, how to use it, and what you should know. I love spending time with my family and sharing stories of the day with them.